The field of natural language processing (NLP) has seen great advancements in recent years, and deep learning models like ChatGPT and BERT have played a significant role in this progress.
In this blog, we’ll take a closer look at these two models and understand what sets them apart. ChatGPT vs BERT
Introduction to ChatGPT and BERT
OpenAI’s GPT-3, or Generative Pretrained Transformer 3, is a large language model trained on a diverse range of internet text. One of its variants, ChatGPT, is specifically trained for conversational AI tasks like text-based dialogue, question-answering, and text generation.
Google’s BERT, on the other hand, stands for Bidirectional Encoder Representations from Transformers, and it is trained on a masked language modeling task. This makes it suitable for a wide range of NLP tasks, including text classification, named entity recognition, and question-answering.
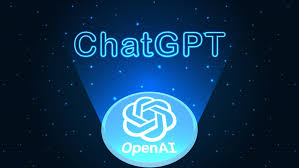
Differences between ChatGPT and BERT
The primary difference between ChatGPT and BERT lies in their training objectives and the tasks they are optimized for. While ChatGPT is trained specifically for conversational AI tasks, BERT is trained for a broader range of NLP tasks.
Another key difference is the architecture of these models. ChatGPT is based on the transformer architecture, which is commonly used in NLP models, but with a focus on the conversational AI tasks. BERT, on the other hand, uses a bidirectional transformer architecture, which allows it to consider the context from both the left and the right of a token, making it suitable for various NLP tasks.
Finally, it’s also worth mentioning that ChatGPT and BERT have different sizes and computational requirements. ChatGPT is a smaller model, which makes it easier to fine-tune for specific conversational AI tasks, but it may not perform as well on more complex NLP tasks as compared to BERT.
Use cases for ChatGPT and BERT
Given their different training objectives and architectures, ChatGPT and BERT are used for different purposes. ChatGPT is mainly used for conversational AI applications, such as chatbots and virtual assistants. With its ability to generate human-like responses, ChatGPT can be used to create engaging and informative conversations with users.
BERT, on the other hand, is widely used for a range of NLP tasks, such as text classification, named entity recognition, and question-answering. Its bidirectional architecture and ability to understand context make it a powerful tool for tasks that require a deep understanding of text.
BERT has been applied in a variety of industries, including finance, healthcare, and retail, for tasks such as sentiment analysis and document summarization.
Best Use of ChatGPT
- Chatbots: ChatGPT can be used to build chatbots that can answer questions and engage in conversations with users in a natural and human-like manner.
- Text Generation: ChatGPT can be used to generate text based on a given prompt, for example, writing captions for social media posts or composing an email response.
- Question-Answering: ChatGPT can be fine-tuned for question-answering tasks, where it can provide answers to questions based on a given context or document.
Best Use of Bert
- Text Classification: BERT can be used for text classification tasks, such as sentiment analysis or categorizing news articles into different topics.
- Named Entity Recognition: BERT can be used for named entity recognition, where it can identify and extract entities like people, organizations, and locations from a text.
- Question-Answering: BERT can also be used for question-answering tasks, where it can provide answers to questions based on a given context or document.
- Sentence Similarity: BERT can be used to determine the similarity between two sentences, which can be useful for various NLP tasks like document clustering or recommendation systems.
These are just a few examples of the many use cases for ChatGPT and BERT. The choice between these two models will depend on the specific requirements of the task at hand, such as the type of data, the desired performance, and the computational resources available.
Pricing
ChatGPT
OpenAI does not publicly disclose the pricing for its language models like ChatGPT. The cost of using OpenAI’s models will depend on various factors, such as the size of the model, the amount of data processed, and the duration of usage. In general, access to OpenAI’s models is offered through its API, which is a paid service that provides access to the models for specific use cases.
BERT
As for BERT, it is an open-source model, so there is no cost associated with using it. However, the cost of deploying a BERT-based solution will depend on various factors, such as the size of the model, the hardware requirements, and the cost of any additional services required to host and run the model.
It is worth noting that the cost of using these models is not limited to the cost of accessing the models themselves, but also includes the cost of fine-tuning and deploying the models, as well as any ongoing maintenance and support required.
Conclusion
In conclusion, ChatGPT and BERT are both powerful language models developed by OpenAI and Google, respectively. While ChatGPT is optimized for conversational AI tasks, BERT is optimized for a wider range of NLP tasks. Both models have their own strengths and weaknesses, and the choice between the two will ultimately depend on the specific requirements of the task at hand.